The current framework of reinforcement learning is mainly based on a single objective performance optimization, that is maximizing the expected returns based on scalar rewards that come either from univariate environment response or from a weighted aggregation of a multivariate response.
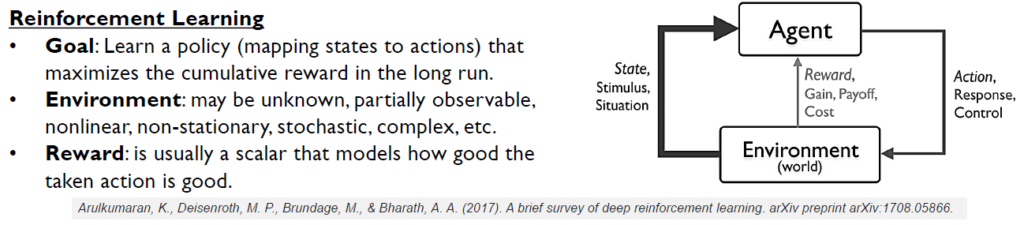
If the problem’s environment is complex, with huge states and actions spaces where the mapping between these two spaces is not necessarily linear, Deep Learning becomes an interesting alternative with end-to-end learning capabilities, even if the data is tabular.
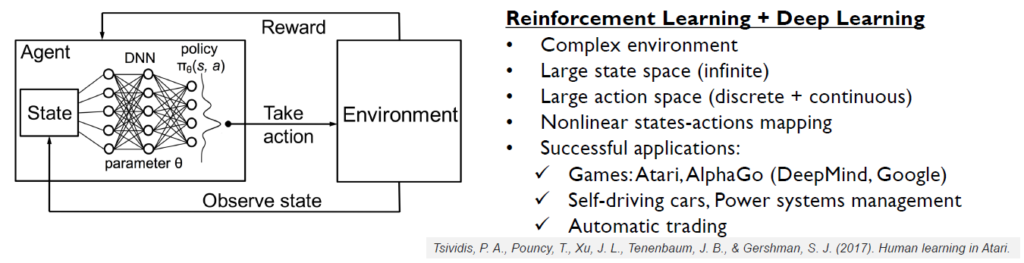
In most of the real-world decision making problems, tradeoffs among multiple conflicting objectives (or goals) that have different order of magnitude, measurement units and business specific contexts related to the problem being solved (i.e. costs, lead time, quality of service, profits, etc.) must be carefully analyzed.
The aggregation of RL sub-rewards to get a scalar reward assumes a perfect knowledge about the decision maker preferences and the way she perceives the importance of each objective.
In ATM CASHVISION®, we designed and implemented a proprietary Multiobjective Deep Reinforcement Learning engine to tackle one of the hardest planning problems faced by banker and cash transportation companies, that is, The ATM Cash Replenishment Optimization and Planning Problem.
Stay tuned to know more about the benefits of our approach !